Data Science – cross-sectional topic
Our efforts of studying and monitoring different materials and systems at all levels, from the scale of single atoms to the level of entire cities, generate large volumes of data. Even more so as digitization, data acquisition systems and simulations significantly increase the amount of data available in industry and in academia. At the same time, the complexity of the data grows due to a variety of different formats, semantics and quality. Handling and interpreting these large sets of data is a tremendous challenge. At the same time, it offers scientists at Empa unique opportunities of learning more about the materials and systems. Thus all aspects linked to the handling and interpretation of large data volumes are encompassed by the cross-sectional topic Data Science.
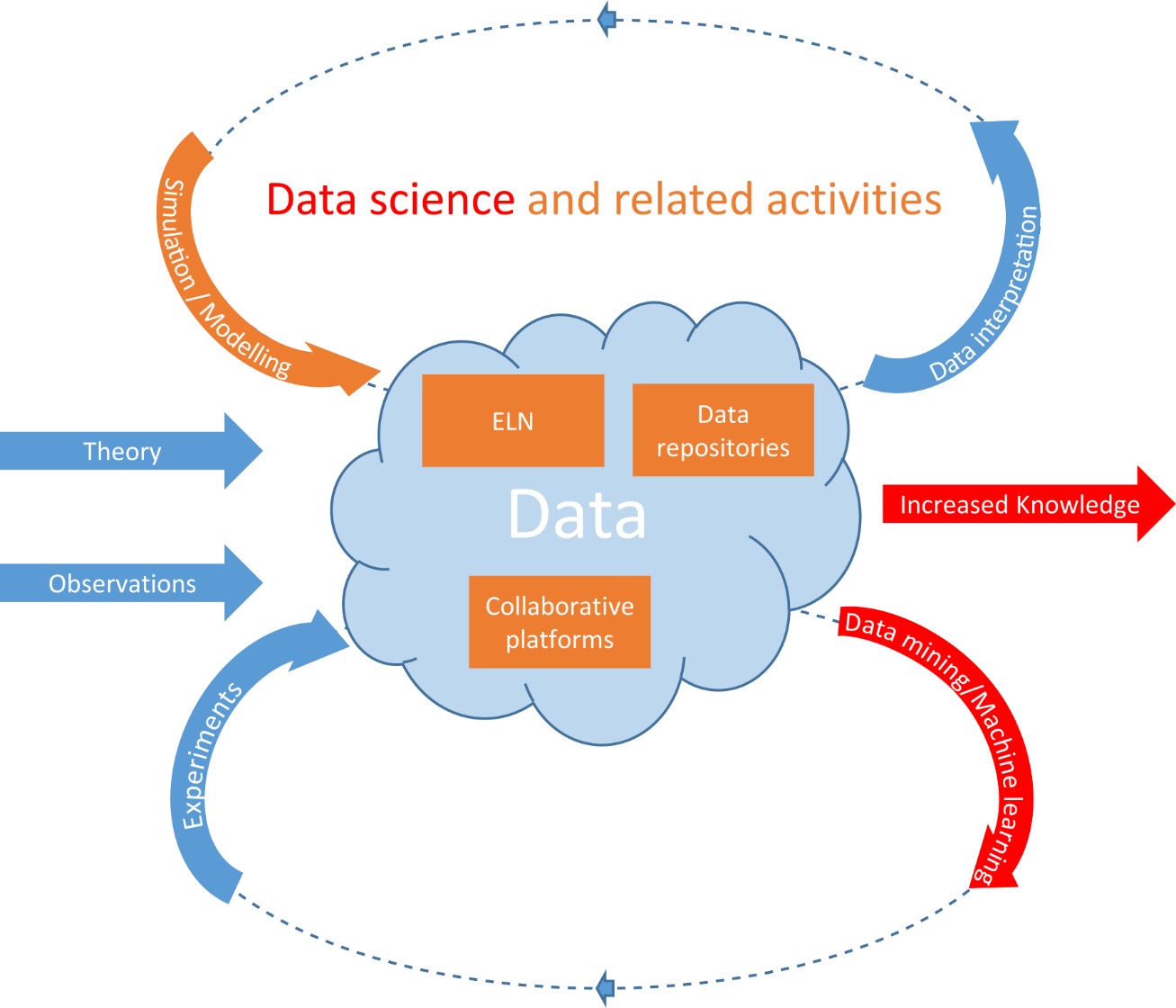
The goal of this cross sectional topic is to develop technologies to convert available data into valuable information and thus gain a comprehensive understanding and extract knowledge from it. This is where methods such as machine learning come into play. Machine learning techniques are valid candidates to overcome major challenges related to the statistical analysis of complex systems. These data-driven approaches are able to find highly complex and non-linear patterns in data of different types and sources and automatically create models that can be applied for detection, classification, regression, or forecasting. Machine learning tools, particularly deep learning based on artificial neural networks, represent key enablers for empowering material scientists and engineers to accelerate the development of novel materials and processes. One of the goals of using these approaches in the field of materials science is to achieve high-throughput identification and quantification of essential features along the process-structure-property-performance chain.
A prerequisite of data science is that data is available and accessible. Thus ways and tools to manage data and to make data easily accessible is an additional activity covered in this cross-sectional topic. This aspect encompasses tools such as collaborative platforms, data repositories as well as electronic lab notebooks. This is also highly relevant in the context of open science.
Finally simulation and modelling activities are also core activity within this cross-sectional topic. Data-driven machine learning and traditional constitutive model-based simulation tools can complement one another leading to highly reliant and efficient hybrid models. Such hybrid approaches can be very efficient in the development of new functional materials. Moreover, modelling and simulation approaches highly profit from good data management approaches, both to handle the large volumes of data they produce and to make reliable data for validation available and accessible.
Dr. Sandra Galmarini
PhD Materials Science_ Atomistic Simulation of cementitious systems, EPFL
-
Share